In the age of information, data has become the currency of modern business. Data driven marketing is no longer a buzzword but a fundamental strategy for organizations looking to thrive in today’s competitive landscape. Central to this approach is the role of analytics in informing marketing decisions. In this comprehensive guide, we will explore the crucial role of analytics in data driven marketing decision making and how organizations can leverage data to drive more effective and personalized marketing campaigns.
Understanding Data Driven Marketing
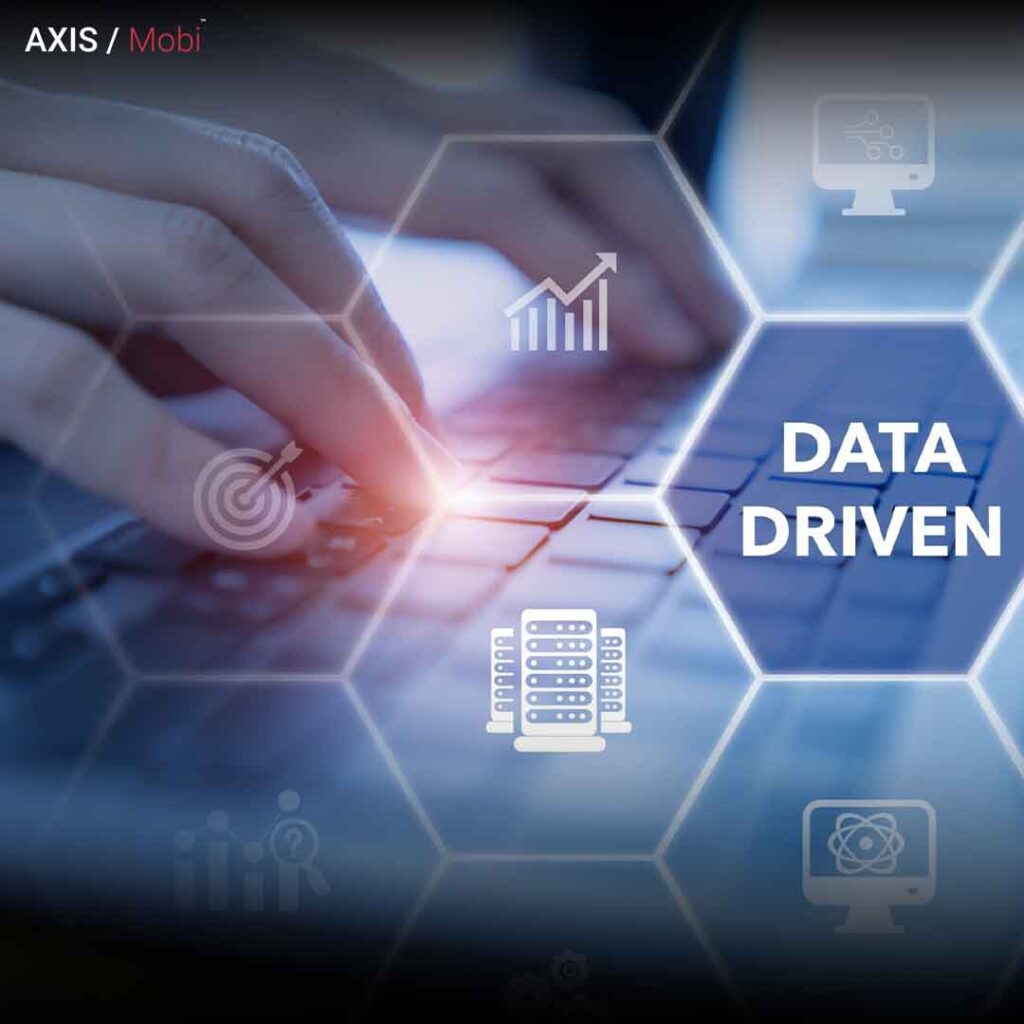
Data driven marketing is a strategic approach that uses data and analytics to guide marketing decisions and campaigns. It involves the collection, analysis, and interpretation of data from various sources to gain insights into customer behavior, preferences, and trends. These insights are then used to inform marketing strategies, enabling businesses to deliver personalized, relevant, and effective campaigns.
Key Principles of Data Driven Marketing
- Data Collection: Gathering data from diverse sources, including websites, social media, email campaigns, customer surveys, and more.
- Data Analysis: Employing advanced analytics tools and techniques to process and interpret the collected data, uncovering valuable insights.
- Personalization: Tailoring marketing messages and content to individual customers based on their behavior, preferences, and demographics.
- Continuous Optimization: Iteratively improving marketing campaigns based on data, feedback, and performance metrics.
- Segmentation: Dividing the audience into segments based on shared characteristics or behavior patterns to target them more effectively.
The Role of Analytics in Data Driven Marketing Decision Making
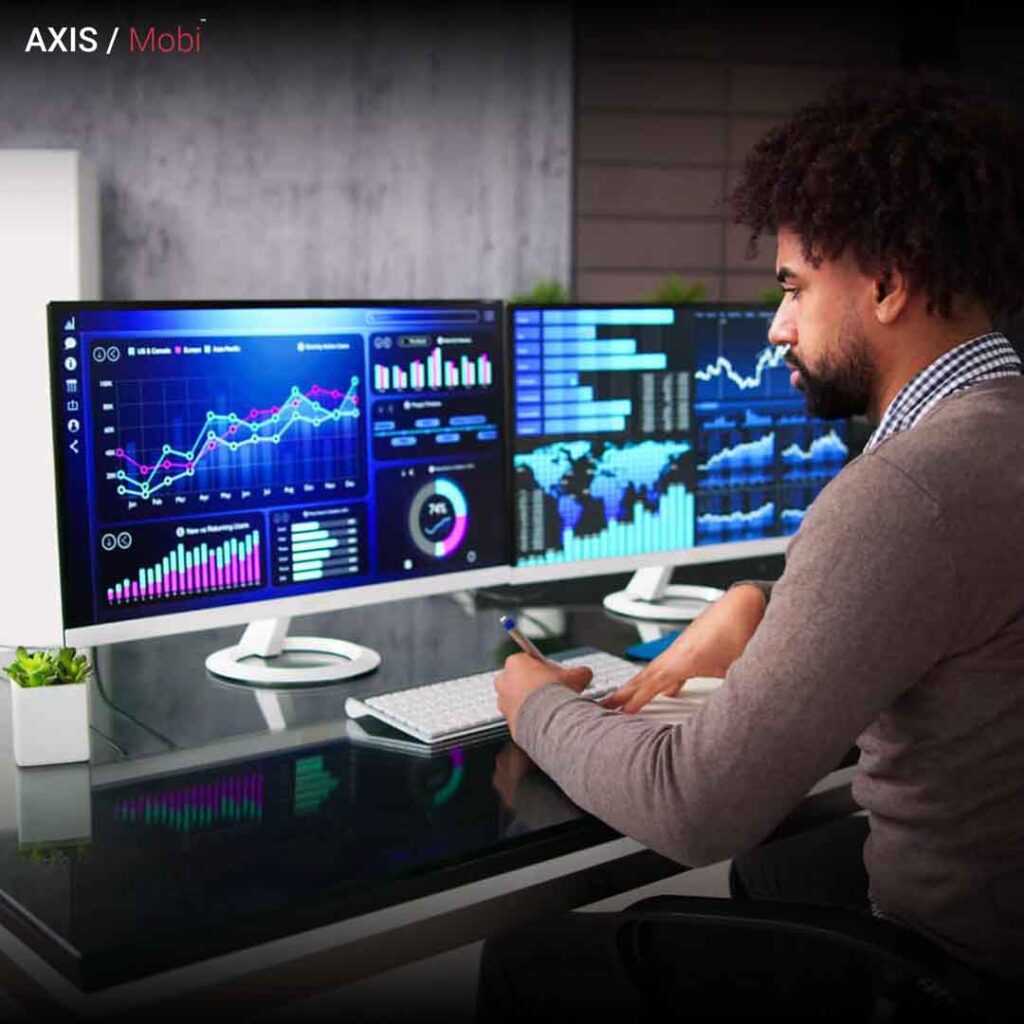
Analytics plays a pivotal role in every phase of the data driven marketing process. Let’s explore how analytics contributes to informed decision making at each stage:
1. Data Collection and Integration
Challenge: Data often resides in disparate sources, making it challenging to create a comprehensive view of the customer.
Role of Analytics: Analytics tools can help organizations collect, clean, and integrate data from various sources. Data warehouses and data lakes are used to store and organize data for analysis. Analytics also aids in setting up automated data pipelines to ensure that data flows smoothly from source to storage.
2. Customer Segmentation
Challenge: To deliver personalized marketing, businesses need to segment their audience effectively.
Role of Analytics: Analytics tools and techniques, such as clustering algorithms, help organizations segment their customer base. By analyzing customer data, businesses can identify common traits, behaviors, and preferences within their audience. These insights lead to more accurate segmentation, enabling tailored marketing strategies for each group.
3. Personalized Content
Challenge: Creating content that resonates with individual customers requires deep insights.
Role of Analytics: Analytics informs content personalization by providing data driven insights into what types of content different segments of the audience find engaging. A/B testing and content performance analysis help determine which content variations are most effective, allowing for the creation of highly personalized content journeys.
4. Data Driven Campaigns
Challenge: Optimizing marketing campaigns and strategies demands real-time insights.
Role of Analytics: Real-time analytics dashboards provide marketers with up-to-the-minute data on campaign performance. These dashboards allow marketers to track key performance indicators (KPIs), identify trends, and make necessary adjustments on the fly. Predictive analytics also aid in forecasting campaign outcomes and optimizing ad spend.
5. Measurement and Analysis
Challenge: Measuring the effectiveness of marketing efforts requires comprehensive data analysis.
Role of Analytics: Analytics tools are essential for measuring and analyzing marketing performance. By evaluating metrics such as conversion rates, click-through rates, customer acquisition costs, and customer lifetime value, organizations gain insights into the impact of their marketing initiatives. Attribution modeling helps attribute conversions and sales to specific marketing touchpoints, allowing for more accurate ROI assessment.
6. Continuous Optimization
Challenge: Marketing strategies must evolve based on data driven insights.
Role of Analytics: Analytics provides the insights needed for continuous optimization. Marketers can use A/B testing, multivariate testing, and other techniques to compare different campaign variations and identify which elements drive better results. Analytics also aids in identifying trends and shifts in customer behavior, enabling organizations to adjust their strategies accordingly.
The Power of Advanced Analytics in Data Driven Marketing
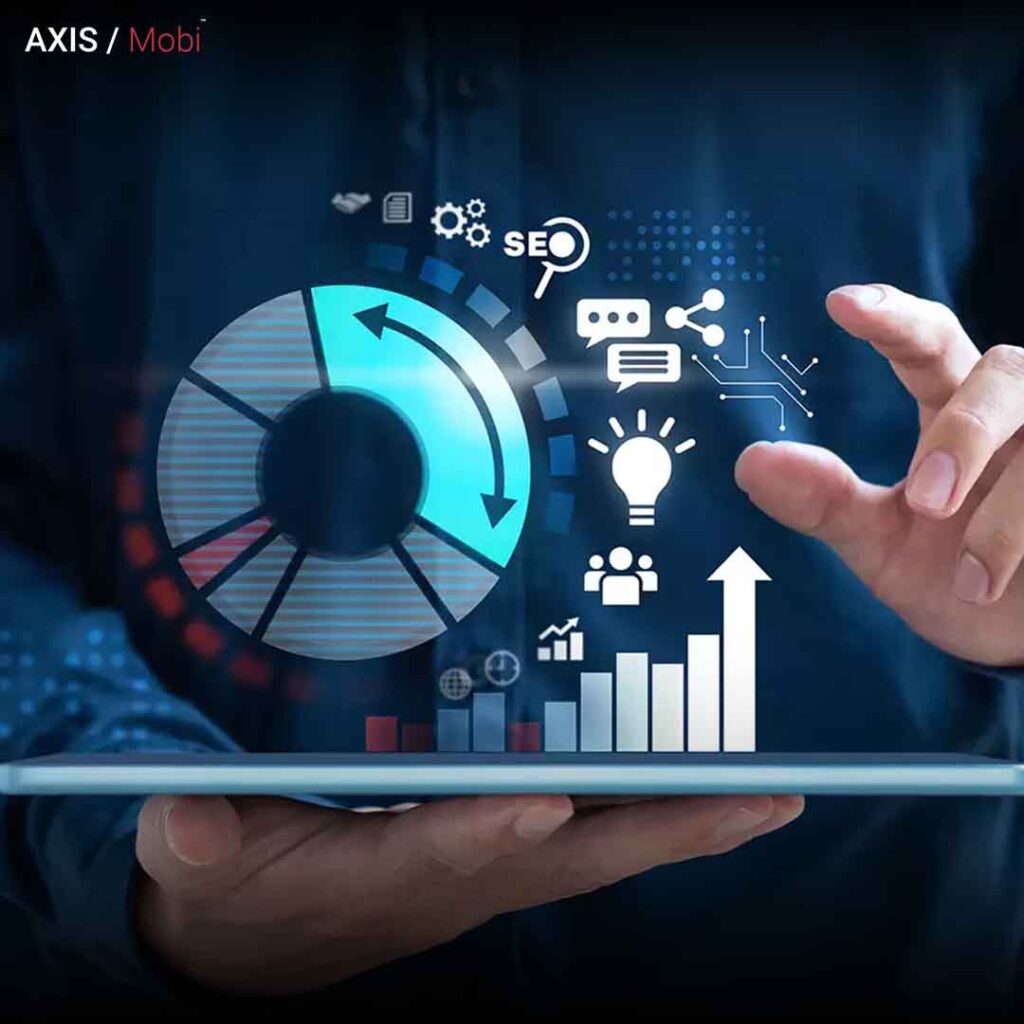
While basic analytics play a crucial role in data driven marketing, advanced analytics techniques further enhance decision-making capabilities:
1. Predictive Analytics
Predictive analytics uses historical data and machine learning algorithms to forecast future events or outcomes. In data driven marketing, predictive analytics can help organizations:
- Predict customer behavior, such as purchase intent or churn likelihood.
- Anticipate trends and emerging market opportunities.
- Optimize marketing strategies by allocating resources to high-converting channels and audiences.
2. Customer Lifetime Value (CLV) Analysis
CLV analysis calculates the total value a customer is expected to generate throughout their relationship with a business. This metric helps organizations:
- Identify high-value customers and tailor marketing efforts to retain them.
- Determine the appropriate customer acquisition costs based on expected lifetime value.
- Prioritize customer segments for personalized marketing based on their potential CLV.
3. Natural Language Processing (NLP)
NLP is a branch of artificial intelligence that focuses on the interaction between humans and computers through natural language. In data driven marketing, NLP can:
- Analyze customer reviews, social media conversations, and feedback to gain qualitative insights.
- Monitor sentiment analysis to gauge public sentiment about products or brands.
- Generate content and recommendations based on customer inquiries and interactions.
4. Attribution Modeling
Attribution modeling assigns value to each marketing touchpoint in a customer’s journey. It helps organizations:
- Understand the impact of various marketing channels and touchpoints on conversions.
- Allocate marketing budgets more effectively by identifying high-impact touchpoints.
- Optimize marketing strategies by adjusting investments in channels that drive the most conversions.
Overcoming Challenges in Implementing Analytics
While analytics is a critical component of data driven marketing, organizations may face challenges in implementing and maximizing its potential:
1. Skill Gap
Challenge: Finding and retaining skilled data analysts and data scientists can be difficult.
Solution: Invest in training and development programs to build data analytics capabilities within your organization. Consider outsourcing or partnering with agencies that specialize in data analytics.
2. Data Privacy and Compliance
Challenge: Stricter data privacy regulations, such as GDPR and CCPA, require businesses to handle customer data with care and ensure compliance.
Solution: Develop and enforce strict data privacy and compliance policies. Implement consent management tools to ensure that customer data is collected and used in accordance with regulations. Educate employees on data privacy best practices.
3. Resource Constraints
Challenge: Implementing analytics solutions can be resource-intensive.
Solution: Prioritize and allocate resources strategically. Consider cloud-based analytics solutions that offer scalability without the need for significant upfront investment in infrastructure.
4. Data Quality
Challenge: Inaccurate or incomplete data can lead to flawed insights and ineffective marketing efforts.
Solution: Regularly audit and cleanse data to ensure accuracy. Implement data validation processes and invest in data quality tools. Establish data governance practices to maintain data integrity.
5. Algorithm Bias
Challenge: Machine learning algorithms used for personalization may introduce bias, leading to unintended consequences.
Solution: Regularly audit and fine-tune algorithms to identify and mitigate bias. Involve diverse teams in algorithm development to minimize bias and ensure fairness.
Conclusion
Analytics is the backbone of data driven marketing decision making. By leveraging analytics tools and techniques, organizations can collect, integrate, and analyze data to gain valuable insights into customer behavior and preferences. These insights inform marketing strategies, enabling businesses to deliver personalized, relevant, and effective campaigns.
From data collection and segmentation to content personalization, campaign optimization, and performance measurement, analytics guides every aspect of data driven marketing. Advanced analytics techniques, such as predictive analytics, CLV analysis, NLP, and attribution modeling, further enhance decision-making capabilities.
As organizations continue to embrace data driven marketing, it is essential to overcome challenges related to skill gaps, data privacy, resource constraints, data quality, and algorithm bias. By doing so, businesses can unlock the full potential of analytics and drive more successful and personalized marketing campaigns. In an era where data reigns supreme, organizations that harness the power of analytics will lead the way in delivering exceptional customer experiences and achieving marketing success. Data driven marketing is not just a strategy; it’s the future of marketing, and analytics is the compass that guides the way.