In today’s data-driven world, businesses and organizations are inundated with vast amounts of data. This data, often referred to as Big Data, comes from various sources such as social media, IoT devices, sensors, and customer interactions. While the sheer volume of data can be overwhelming, it also presents a goldmine of opportunities. The key to unlocking the potential of Big Data lies in Big Data Analytics – a powerful approach that can turn raw data into actionable insights. In this comprehensive guide, we will explore the world of Big Data Analytics, its significance, technologies, challenges, and how organizations can leverage it to make informed, data-driven decisions.
Understanding Big Data Analytics

Before we dive deeper into Big Data Analytics, let’s first define what it is:
Big Data Analytics is the process of examining and interpreting large datasets to uncover hidden patterns, correlations, and insights. It involves the use of advanced analytical techniques and technologies to extract valuable information from massive volumes of structured and unstructured data. The goal is to gain a deeper understanding of trends, customer behavior, and other critical factors that can inform decision-making.
Key Components of Big Data Analytics:
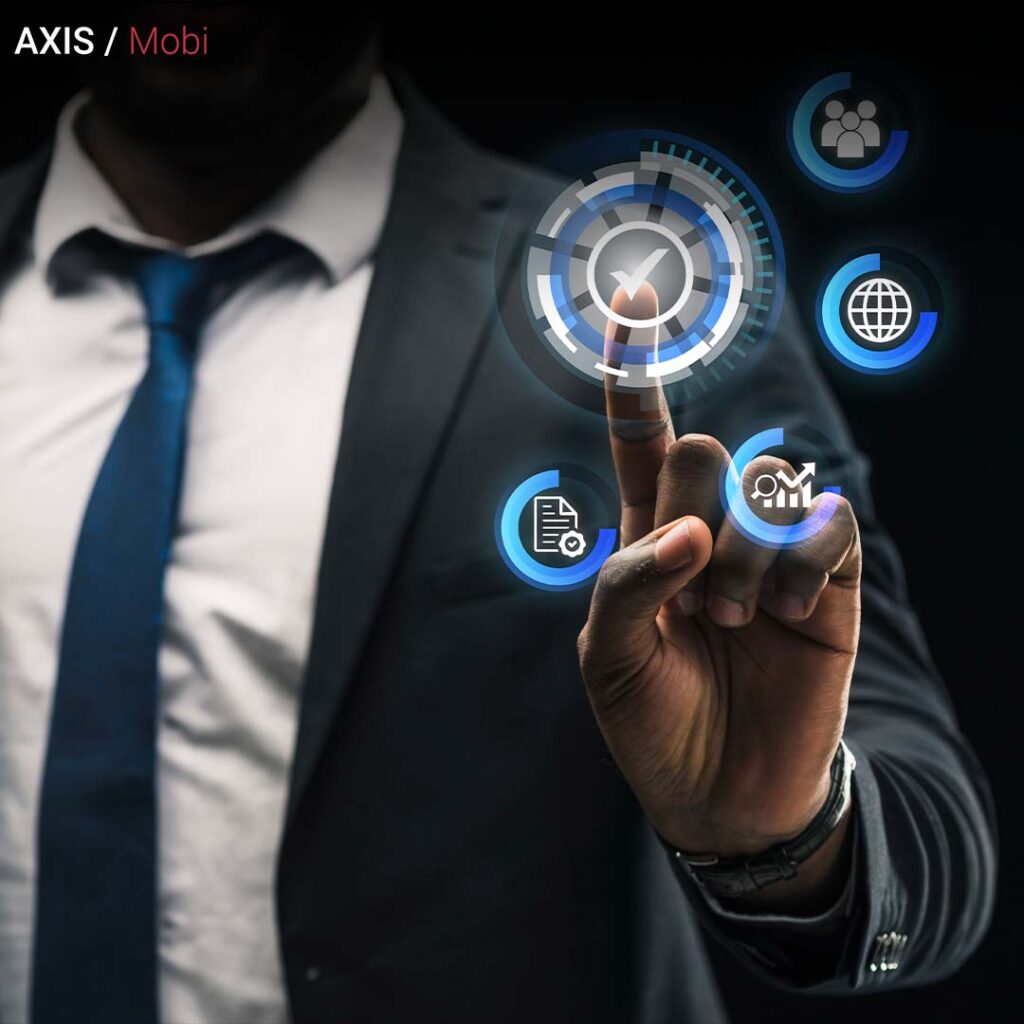
- Data Collection: Gathering data from various sources, including databases, social media, sensors, and more.
- Data Storage: Storing and managing data efficiently, often in distributed databases or data lakes.
- Data Processing: Employing techniques like data cleaning, transformation, and enrichment to prepare data for analysis.
- Data Analysis: Applying statistical, machine learning, and AI algorithms to identify patterns and insights.
- Data Visualization: Presenting findings through charts, graphs, and dashboards for easy interpretation.
The Significance of Big Data Analytics
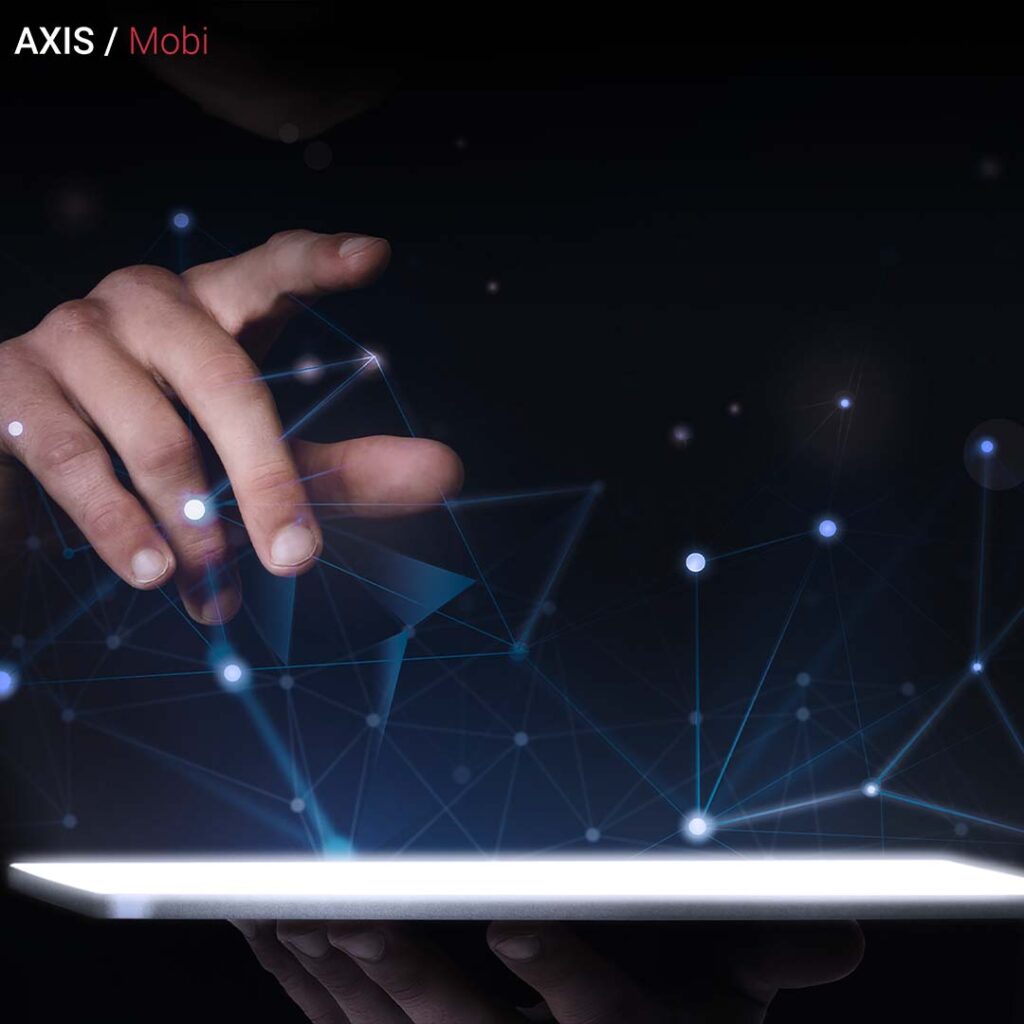
Why is Big Data Analytics so important in today’s business landscape? Here are some compelling reasons:
1. Informed Decision-Making
Big Data Analytics empowers organizations to make data-driven decisions. By analyzing large datasets, decision-makers gain insights that can inform strategies, product development, and marketing campaigns.
2. Improved Efficiency
Analyzing data can reveal inefficiencies in processes and operations. By addressing these inefficiencies, organizations can streamline workflows and reduce costs.
3. Enhanced Customer Experience
Understanding customer behavior through data analysis allows organizations to tailor products and services to meet customer needs and preferences. This leads to improved customer satisfaction and loyalty.
4. Competitive Advantage
Organizations that effectively leverage Big Data Analytics gain a competitive edge. They can identify emerging market trends, respond to customer demands more swiftly, and stay ahead of competitors.
5. Risk Management
Data analysis can identify potential risks and threats to an organization. By identifying these risks early, organizations can take proactive measures to mitigate them.
The Technologies Behind Big Data Analytics
Big Data Analytics relies on a combination of technologies to process, analyze, and visualize data. Here are some of the key technologies and tools:
1. Hadoop
Hadoop is an open-source framework for distributed storage and processing of large datasets. It allows organizations to store and process massive amounts of data across clusters of commodity hardware.
2. Apache Spark
Apache Spark is a powerful data processing framework that provides fast in-memory data processing capabilities. It’s known for its speed and ease of use in processing large datasets.
3. Machine Learning and AI
Machine learning algorithms and artificial intelligence are essential components of Big Data Analytics. These technologies enable predictive modeling, clustering, classification, and more.
4. Data Visualization Tools
Tools like Tableau, Power BI, and QlikView allow organizations to create interactive and visually appealing dashboards and reports to present data insights.
5. NoSQL Databases
NoSQL databases like MongoDB and Cassandra are used to store and manage unstructured or semi-structured data, which is common in Big Data scenarios.
Challenges in Big Data Analytics
While Big Data Analytics offers immense potential, it also comes with challenges that organizations must overcome:
1. Data Volume
Managing and processing massive volumes of data can be resource-intensive. Organizations need robust infrastructure and storage solutions to handle these volumes effectively.
2. Data Variety
Big Data is often diverse, comprising structured and unstructured data from various sources. Integrating and processing such diverse data types can be complex.
3. Data Velocity
Data streams in at high speeds in real-time applications. Analyzing and making decisions based on rapidly changing data requires advanced processing capabilities.
4. Data Quality
Data quality is crucial for accurate analysis. Inaccurate or incomplete data can lead to incorrect conclusions and decisions.
5. Data Security and Privacy
With the increase in data breaches, organizations must prioritize data security and comply with data protection regulations like GDPR and CCPA.
Applications of Big Data Analytics
Big Data Analytics finds applications in various industries and sectors. Let’s explore some real-world examples:
1. Healthcare
Predictive Analytics for Disease Outbreaks: Analyzing health data can help predict disease outbreaks and allocate resources more effectively.
2. Retail
Personalized Recommendations: E-commerce platforms use Big Data Analytics to offer personalized product recommendations based on a customer’s browsing and purchase history.
3. Finance
Fraud Detection: Financial institutions use real-time analytics to detect fraudulent transactions and prevent financial losses.
4. Manufacturing
Predictive Maintenance: Manufacturers use data from sensors on machines to predict when maintenance is needed, reducing downtime and maintenance costs.
5. Marketing
Customer Segmentation: Marketers segment customers based on demographic and behavioral data to target them with more relevant advertisements.
6. Transportation
Route Optimization: Transportation companies optimize routes in real-time to save fuel costs and improve delivery times.
7. Energy
Smart Grids: Energy providers use Big Data Analytics to optimize energy distribution, reduce wastage, and respond to fluctuations in demand.
Conclusion
Big Data Analytics has emerged as a game-changer for organizations across industries. It enables data-driven decision-making, improves efficiency, enhances the customer experience, and provides a competitive advantage. While the challenges are real, the rewards of effectively leveraging Big Data Analytics are substantial.
In a world where data is often described as the new oil, organizations that embrace Big Data Analytics are better equipped to navigate the complexities of the digital age. By investing in the right technologies, ensuring data quality, and staying vigilant about data security and privacy, organizations can harness the power of Big Data to drive innovation and success.